Your Partner
in Legal Success
| Reading Time: 11 Minutes
Deployment of Algorithms and AI in Judicial Systems
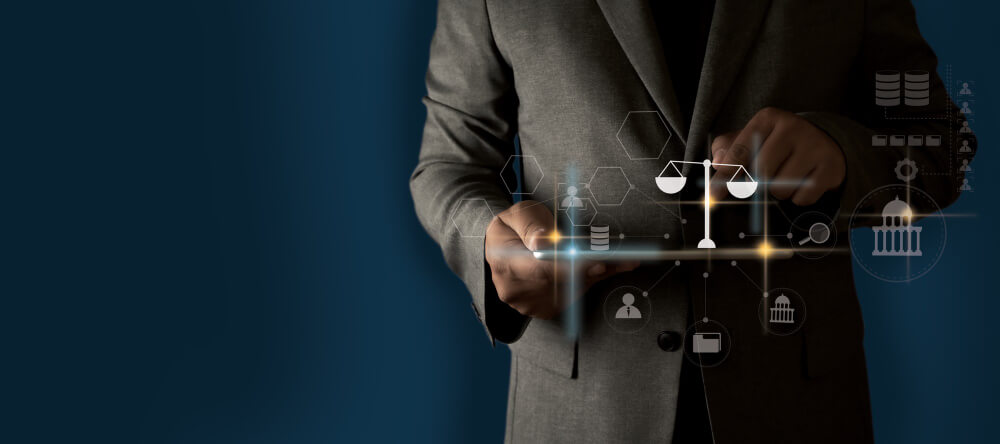
The Deployment of Algorithms and Artificial Intelligence in Judicial Systems
This paper aims to discuss the deployment of algorithms and artificial intelligence in judicial systems and its repercussions on society.
The use of algorithms in judiciary proceedings is not a new concept. Although, the deployment of AI in judicial systems might be a relatively new concept enabled by an enormous amount of data available in our modern-day. These algorithms assess probabilities such as; reoffending, attending court hearings, a risk to society.
They are also employed in pre-trial, probation and parole decisions and even in sentencing decisions. These algorithms have strong proponents and opponents. The main arguments of proponents are the objectivity and efficiency of these tools, whereas opponents believe algorithms are biased and inaccurate in their results. In this discussion, I stand closer to my opponents. While exploiting the benefits of technology will make our lives easier, unfettered reliance on these tools has serious impacts on real human lives.
At present, more than 60 risk assessment tools are being used in the USA.[1] Some parameters used to assign scores to defendants can be categorised as static and dynamic factors. Static factors also referred to as “immutable traits” can include criminal background, gender, other offenders in the family.[2] On the other hand, dynamic factors can include present age, recruitment status or drug/alcohol treatments being undertaken.[3]
One of the most well-known and contradictory risk assessment tools is Correctional Offender Management Profiling for Alternative Sanctions (COMPAS). COMPAS is a fourth-generation risk and needs assessment tool, which is to say it combines dynamic and static risk factors. It is being used across the USA ‘to inform decisions regarding the placement, supervision and case management of offenders.’[4] COMPAS uses a questionnaire consisting of 137 questions to assess the recidivism risk of a defendant.[5]
AI is not flawless, nor the AI-based tools. An issue Julia Angwin et al. had revealed is the racial inequalities of COMPAS assessments. The model classifies black people wrongly as future re-offenders twice the times as white defendants. When questions arise whether these results were because of defendants’ previous crimes or the severity of crimes they committed, ProPublica ran a statistical test dissociating the effect of race, age and gender and still reach at the same wrong detection of black people as high-risk future criminals.[6] There are many examples where white people with more offences in the past were found low risk while a black person with the same offence but none or fewer offences in the past was found high risk by the software.[7]
Moreover, what put Northpointe[8] in a controversial position is its proprietary algorithm. It is a for-profit company, and even though it shares the factors that play a role in reaching a risk rate, it doesn’t disclose the specific calculations and weighing of those factors to the public. We don’t know which question’s answer has affected how much the final assessment.
The rule of law principle requires remembering due process rights laid out in the fifth and fourteenth amendments. Due process gives a person the right to know what charges and what evidence against him/her is brought forward. Frank Pasquale[9] commentates, “A secret risk assessment algorithm that offers a damning score is analogous to evidence offered by an anonymous expert, whom one cannot cross-examine.”[10] In the jurisdictions where the rule of law applies decision-making process is ‘examinable and contestable.’[11] It promotes legal certainty.[12] Legitimate decisions can be provided if the law is accessible, intelligible, clear and predictable.[13]
The opacity of algorithms hinders the rule of law and legitimacy of the decisions. When AI and machine learning are involved in these instruments, the situation may be exacerbated due to the “black box effect” of machine learning (ML). In ML, every new data leads the software to new acknowledgements, classifications, linkages between data points. This may become so complicated that even to the software designer, it is unintelligible and uninterpretable.
In famous Loomis v. Wisconsin, Loomis argued that proprietary portions of COMPAS prevented him from challenging the decision.[14] In Loomis, the court did not accept his arguments regarding the state’s defence in which the judge explained he would come to the same conclusion even without the risk assessment tool but addressed Loomis’s due process concerns in two points.[15] The Supreme Court put forward the ‘limitations and cautions’ sentencing court must behold when utilising COMPAS.
The tool can be a ‘relevant factor’ only in matters:
(1) diverting low-risk prison-bound offenders to a non-prison alternative,
(2) assessing whether an offender can be supervised safely and effectively in the community,
(3) imposing terms and conditions of probation, supervision, and responses to violations.[16]
Secondly, the court significantly acknowledged that a Presentence Investigation Report (“PSI”) containing a COMPAS should inform sentencing courts with:
(1) The proprietary nature of COMPAS has been invoked to prevent disclosure of information relating to how factors are weighed or how risk scores are determined.
(2) Because COMPAS risk assessment scores are based on group data, they can identify groups of high-risk offenders — not a particular high-risk individual.
(3) Some studies of COMPAS risk assessment scores have raised questions about whether they disproportionately classify minority offenders as having a higher risk of recidivism.
(4) A COMPAS risk assessment compares defendants to a national sample, but no cross-validation study for a Wisconsin population has yet been completed. Risk assessment tools must be constantly monitored and re-normed for accuracy due to changing populations and subpopulations.
(5) COMPAS was not developed for use at sentencing but was intended for the Department of Corrections to make determinations regarding treatment, supervision, and parole.[17]
Opaque risk assessment tools lead to unaccountable decisions. Eventually, it occurs to one that judges can be questioned for their disparate acts, but an algorithm subtly injected with bias may never be criticised.[18] Transparency and accountability are mandatory for the confident use of AI. Therefore, people should be assured that an independent body oversees these life-impacting tools and that this entity scrutinises their inner workings and decision-making processes.
One of the goals sought from using risk assessment tools is reducing recidivism. American Bar Association is one of the organisations which prompted the use of algorithms in states.[19] However, it also highlighted the abovementioned labelling of low-risk individuals as a high-risk problem that may be destructive to the individual’s rehabilitation efforts and raise rather than reduce recidivism.[20]
Objectivity and accuracy are other arguments of the actuarial risk assessment tools’ proponents. While many tools are being used across the US, it is hard to say they are validated adequately. In the US, 48 per cent pretrial assessment tools have never been validated.[21] According to some experts, validity is dampened by the importance given to accuracy.[22]
Machine learning is applied in the COMPAS risk model. ProPublica examined and found out that the algorithm is not reliable in predicting violent crimes with only a 20 per cent accuracy rate.[23] When it comes to misdemeanours, the algorithm was moderately more accurate, with 61 per cent.[24]
Furthermore, Julia Dressel and Hany Farid conducted a study and found out that laypeople with no criminal law expertise can be as accurate as COMPAS.[25] Another discrepancy in COMPAS is false-positive rates. The program has a false-positive rate of 40.4% for black offenders and 25.4% for white offenders, which is a considerable disparity between the two races. The bias proves that even though race is not a parameter point in COMPAS, some data “act as proxies for racial data.”[26]
There is another concerning aspect related to this discussion. According to the research by Corbett-Davies et al., black defendants have a higher reoffending rate at 51%, while white defendants have a 39% rate of reoffending in Broward County, Florida. However, these rates are akin to countrywide ratios.[27] When these data collected from different jurisdictions are entered into the software program, it may cause bias and discrimination to get mechanical and automated.
There are some other criticisms for the use of risk assessment tools in judicial procedures. For one, employing a pre-trial tool to sentencing decisions. This is criticised for the sentencing decision having multiple goals while the tool only weighs the recidivism risk.[28] Thus the decisions out of that software may not satisfy sentencing objectives such as “individual retribution, rehabilitation, deterrence, and incapacitation.”[29] Secondly, a tool created for pre-trial context and validated does not mean it is valid in sentencing procedures. A tool modelled to scale recidivism and flight risk has no relevance in a sentencing hearing.[30]
Some scholars believe that risk assessment tools impend individualised justice and presumption of innocence which are fundamental norms of the common law. Actuarial instruments compare a defendant’s information with the group data it already has and forms an estimation of the defendant’s risk level, not with a tailored evaluation for that particular person. It is hard to get a result that will fit the crime and the offender’s unique capabilities from such a system.
Moreover, the evolution of risk assessment tools transformed the criminal justice environment to where potential harms, risk, pre-emption, precaution and risk prevention concepts sway.[31] When these notions are prioritised over real threats, it depreciates the presumption of innocence. Also, in bail decisions, false positives -defendants who are mistakenly rated as high-risk while they are low-risk- may compromise the presumption of innocence.[32]
Besides, the opaque nature of algorithms undermines the burden of proof on the prosecution when there is no explanation for the risk assessment tool calculations.[33] Therefore no explanation exists for the assessments either. One other concern is that deskilling. Deployment of AI in judicial processes may gradually cause judges, lawyers or legal experts to become deskilled.[34] Not doing the same examinations, inquisitions, analyses, not applying specific tests, or not referring to case law may ultimately result in downgrading skills. By the time, even if the program gets distorted, they may not even be able to recognise it.[35]
Some scholars resemble this situation to an automatic pilot mode in airplanes,[36] although it shouldn’t be easy to make this resemblance. When judges make decisions, they take into account individualised justice, equal treatment of equals, the punishment fits the crime, the purposes of sentencing; “punishment, deterrence, community protection, rehabilitation, accountability, denouncement and recognition of the harm inflicted on the victim and the community,”[37] empathy, sympathy, and humane sentiments. Can all these principles be translated to algorithmic instruments and AI, or do we trade-off these fundamental values of the justice system for the sake of numerical precisions and mechanical consistencies -notwithstanding the fact that these are sceptical-?
I would like to quote from Hildebrandt at this point:
“Artificial intelligence similarly depends on the processing of information (in the form of digital data points). Neither in the case of organisms nor in the case of intelligent machines does information necessarily imply the attribution of meaning, as it may in the case of humans. Machines work with signs, they do not speak the human language; they ‘live’ in a ‘world’ made of hardware and software, data, and code. Their perception is limited to machine-readable data, their cognition is based on computation and manipulation of signs, not on generating meaning (though we may attribute meaning to their output and/or their behaviour).”[38]
Risk assessments attributed to machines have been at human discretion, and intuition fettered by-laws, guidelines, jurisprudence and legal principles for centuries. In judicial procedures, what is at stake is human lives. Who is suffering is human, the whole process is very human. “Diminution of judicial human evaluation”[39] and ceding it sheerly to artificial intelligence is not conscientious at the least.
With all these concerns being said, as Benjamin Alarie foresees, ever-growing technology will also dominate the legal field.[40] We need some baselines, guidelines, ethics to use AI in judicial procedures. Partnership on AI has a comprehensive requirement list for risk assessment tools in its Report on Algorithmic Risk Assessment Tools in The U.S. Criminal Justice System.[41] These requirements emphasised bias mitigation, easy interpretability, training for the users, contestability, openness, and auditing of these tools.[42] As listed in the report, we need accountability, transparency, and fairness in risk assessment tools to exploit the benefits of these tools.
Alongside the requirements, we should be aware of some ancillary issues such as who designs the AI, which funds the designing, does the tool reinforce the interests and concerns of those who funded it?[43] Additionally, the efficacy of such tools will be proportionate to the quality of training the software and training data. Lawyers and legal authorities should keep themselves up-to-date and make sure it is well adjusted with the rule of law and our fundamental legal values.[44]
Technological advancement and the sum of work AI can handle are astounding, but this is not the end of the story. According to the experts’ Partnership on AI consulted, current risk assessment tools are not ready to make decisions on defendants’ detention or liberty without a physical individualised hearing.[45] Remember Tay of Microsoft, how she became a racist and misogynistic robot in 2/3 of a day, then what could our hundred years of history full of sedecidelation of human rights teach AI-based tools? These tools should not be used without validation, transparency, accountability, accuracy, fairness and auditing. Otherwise, “it may propagate the very bias so many people put their lives on line to fight.”[46]
All in all, what I think we should keep at someplace in our minds is beautifully articulated by Zeynep Tufekci: “Being fully efficient, always doing what you’re told, always doing what, you’ve programmed is not always the most human thing. Sometimes it’s disobeying, sometimes it’s saying “No, I’m not gonna do this,” right? And if you automate everything, so it always does what it’s supposed to do, sometimes that can lead to very inhuman things.”[47]
Article Keywords: Deployment of Algorithms and AI in Judicial Systems, Deployment of Algorithms and Artificial Intelligence in Judicial Systems, AI in judicial systems, Artificial Intelligence in Judicial Systems, Algorithms and AI in Judicial Systems, Algorithms and Artificial Intelligence in Judicial Systems, Machine Learning, Judicial Systems, Artificial Intelligence, AI, Algorithms in judicial systems.
Contact us if you find any errors in the article.
References
- [1] Rahnama, Kia, Science and Ethics of Algorithms in the Courtroom (May 10, 2019). Journal of Law, Technology and Policy, Vol. 2019, No. 1, 2019, p. 174, Available at SSRN: <https://ssrn.com/abstract=3386309>.
- [2] Kehl, Danielle, Priscilla Guo, and Samuel Kessler. 2017. Algorithms in the Criminal Justice System: Assessing the Use of Risk Assessments in Sentencing. Responsive Communities Initiative, Berkman Klein Center for Internet & Society, Harvard Law School, p.9, Available at <http://nrs.harvard.edu/urn-3:HUL.InstRepos:33746041>.
- [3] Id.
- [4] Northpointe, Practitioner’s Guide to COMPAS Core, March 19 2015, p. 1, Available at <https://assets.documentcloud.org/documents/2840784/Practitioner-s-Guide-to-COMPAS-Core.pdf>.
- [5] Julia Dressel & Hany Farid, The Accuracy, Fairness, and Limits of Predicting Recidivism, 4 SCI. ADVANCES (2018), Available at <https://advances.sciencemag.org/content/4/1/eaao5580>.
- [6] Julia Angwin, Jeff Larson, Surya Mattu and Lauren Kirchner, Machine Bias, ProPublica, May 23 2016, <https://www.propublica.org/article/machine-bias-risk-assessments-in-criminal-sentencing>, 77 per cent more likely for a violent crime and 45 per cent more likely for any crime.
- [7] See generally Id.
- [8] The company created COMPAS. Now “Equivant”
- [9] Professor of law at Brooklyn Law School and the author of The Black Box Society and New Laws of Robotics books.
- [10] Frank Pasquale, Secret Algorithms Threaten the Rule of Law, MIT Technology Review, June 1 2017, <https://www.technologyreview.com/2017/06/01/151447/secret-algorithms-threaten-the-rule-of-law/>.
- [11] McKay, Carolyn, Predicting Risk in Criminal Procedure: Actuarial Tools, Algorithms, AI and Judicial Decision-Making (November 27, 2019). McKay, C., ‘Predicting Risk in Criminal Procedure: Actuarial Tools, Algorithms, AI and Judicial Decision-Making’, Current Issues in Criminal Justice, 2019 (Forthcoming), Sydney Law School Research Paper No. 19/67, p. 15, citing from Oswald 2018, Available at SSRN: <https://ssrn.com/abstract=3494076> or <http://dx.doi.org/10.2139/ssrn.3494076>.
- [12] Id. citing from Hildebrandt 2018.
- [13] Id. citing from Gordon 2017: 2 quoting Lord Bingham 2006.
- [14]Rizer, Arthur and Watney, Caleb, Artificial Intelligence Can Make Our Jail System More Efficient, Equitable and Just (February 24, 2018), p.18, Available at SSRN: <https://ssrn.com/abstract=3129576> or <http://dx.doi.org/10.2139/ssrn.3129576>.
- [15] Id.
- [16] No. 2015AP157-CR. 881 N.W.2d 749 (2016) 2016 WI 68, ¶ 88 <https://www.courts.ca.gov/documents/BTB24-2L-3.pdf>
- [17] Id. at ¶ 100.
- [18] Supra note 11, p. 16.
- [19] Id. at ¶ 2.
- [20] Id.
- [21] Supra note 13, p.10, citing from CYNTHIA A. MAMALIAN, PRETRIAL JUSTICE INST., STATE OF THE SCIENCE OF PRETRIAL RISK ASSESSMENT 21–22 (2011)
- [22] Bagaric, Mirko and Svilar, Jennifer and Bull, Melissa and Hunter, Dan and Stobbs, Nigel, The Solution to the Pervasive Bias and Discrimination in the Criminal Justice: Transparent Artificial Intelligence (March 2, 2021). American Criminal Law Review, Vol. 59, No. 1, p.32, Forthcoming, Available at SSRN: <https://ssrn.com/abstract=3795911>, citing from PARTNERSHIP ON AI, REPORT ON ALGORITHMIC RISK ASSESSMENT TOOLS IN THE U.S. CRIMINAL JUSTICE SYSTEM.
- [23] Julia Angwin, Jeff Larson, Surya Mattu and Lauren Kirchner, Machine Bias, ProPublica, May 23 2016, https://www.propublica.org/article/machine-bias-risk-assessments-in-criminal-sentencing.
- [24] Id.
- [25] See for details, Julia Dressel and Hany Farid Science Advances 17 Jan 2018: Vol. 4, no. 1, eaao5580 DOI: 10.1126/sciadv.aao5580 <https://advances.sciencemag.org/content/4/1/eaao5580>.
- [26] Supra note 18, p. 43.
- [27] Supra note 21, citing from S. Corbett-Davies, E. Pierson, A. Feller, S. Goel, “A computer program used for bail and sentencing decisions was labelled biased against blacks. It’s actually not that clear,” Washington Post, 17 October 2016.
- [28] Supra note 16, at ¶ 3. Since COMPAS is being used for sentencing whereas it was designed to rate recidivism for pre-trial judgments.
- [29] Supra note 2, p. 13, citing from Model Penal Code § 1.02(2).
- [30] PARTNERSHIP ON AI, REPORT ON ALGORITHMIC RISK ASSESSMENT TOOLS IN THE U.S. CRIMINAL JUSTICE SYSTEM, Requirement 3, <https://www.partnershiponai.org/report-on-machine-learning-in-risk-assessment-tools-in-the-u-s-criminal-justice-system/>.
- [31] Supra note 11, p. 4, citing from Brown et al. 2015: 43-44.
- [32] Supra note 2, p. 5, citing from Peter W. Greenwood with Allan Abrahamse, Selective Incapacitation, RAND Corporation (Aug. 1982) (RAND Report).
- [33] Supra note 11, p. 14, citing from Woolmington v DPP [1935] AC 462.
- [34] Mireille Hildebrandt, Law as computation in the era of artificial legal intelligence: Speaking law to the power of statistics, University of Toronto Law Journal, Volume 68, Supplement 1, 2018, University of Toronto Press, p.28, Available at <https://muse-jhu-edu.ezproxy.library.qmul.ac.uk/article/688832>.
- [35] Id. p. 33.
- [36] Supra note 22, p. 4.
- [37] See generally, supra note 11.
- [38] Supra note 33, p. 26
- [39] Supra note 11, p. 1
- [40] Alarie, Benjamin. “The Path of the Law: Towards Legal Singularity.” University of Toronto Law Journal, vol. 66, no. 4, Fall 2016, p. 443-455. HeinOnline, <https://heinonline-org.ezproxy.library.qmul.ac.uk/HOL/P?h=hein.journals/utlj66&i=451>.
- [41] Supra note 30.
- [42] Full list of requirements: Training datasets must measure the intended principles; Bias in statistical models must be measured and mitigated; Tools must not conflate multiple distinct predictions; Predictions and how they are made must be easily interpretable; Tools should produce confidence estimates for their predictions; Users of risk assessment tools must attend pieces of training on the nature and limitations of the tools; Policymakers must ensure that public policy goals are appropriately reflected in these tools; Tool designs, architectures, and training data must be open to research, review, and criticism; Tools must support data retention and reproducibility to enable meaningful contestation and challenges; Jurisdictions must take responsibility for the post-deployment evaluation, monitoring, and auditing of these tools.
- [43] Supra note 33, p. 31.
- [44] Id.
- [45] Supra note 30, Executive Summary.
- [46] Coded Bias movie.
- [47] Zeynep Tufekci, Writer, professor at the University of North Carolina at Chapel Hill. From her speech in the Coded Bias movie.